The Nobel Prize in Chemistry Awarded to Hassabis and Others for Protein Prediction, Marking AI for Science; The Physics Prize is Science for AI
Published: October 9, 2024, 20:31 Source: Weijin Research Shanghai
In 2024, another Nobel Prize was awarded to AI! This time it was the Chemistry Prize!
Half of the award went to David Baker from the University of Washington "for his contributions to computational protein design"; the other half went to Demis Hassabis and John M. Jumper of Google DeepMind "for their contributions to protein structure prediction."
Can you believe that yesterday's winner, Hinton, was a physicist, and today's winner, Hassabis, is a chemist?
More accurately, this award is about proteins—the sophisticated chemical tools of life. Baker accomplished the near-impossible: building entirely new kinds of proteins. Hassabis and Jumper developed an AI model that solved a 50-year-old problem: predicting the complex structures of proteins. These discoveries have tremendous potential and limitless application prospects.
The diversity of life proves the incredible capabilities of proteins as chemical tools. They control and drive all the chemical reactions that are fundamental to life. Proteins also act as hormones, signaling substances, antibodies, and building blocks of various tissues.
"One of the discoveries recognized this year involves the creation of astonishing proteins. The other achieves a 50-year dream: predicting protein structures from amino acid sequences. Both discoveries open up vast possibilities," said Heiner Link, Chairman of the Nobel Chemistry Prize Committee.
Proteins are typically made up of 20 different amino acids, which can be described as the "LEGO blocks" of life. In 2003, Baker successfully used these building blocks to design a new protein completely different from any other protein. Since then, his research team has continuously created imaginative proteins, including those that can be used as drugs, vaccines, nanomaterials, and microsensors.
The second discovery involves predicting protein structures. In proteins, amino acids are linked together in long chains, which fold into three-dimensional structures that are crucial for protein function. Since the 1970s, researchers have been trying to predict protein structures from amino acid sequences, but this work has been notoriously difficult. However, four years ago, there was a breakthrough.
In 2020, Hassabis and Jumper introduced an AI model called AlphaFold2. With this model, they were able to predict the structures of nearly 200 million proteins that researchers had already identified. Since the breakthrough, more than 2 million users from 190 countries have used AlphaFold2. In many scientific applications, researchers can now better understand antibiotic resistance and create enzymes that can break down plastic.
Without proteins, life cannot exist. Now that we can predict protein structures and design our own proteins, this brings great benefits to humanity.
Following yesterday's Nobel Prize in Physics awarded to Hopfield and Hinton for their contributions to AI's fundamental theories, the Chemistry Prize was awarded to Baker, Hassabis, and Jumper for using AI to design and predict protein structures. The Physics Prize is Science for AI, while the Chemistry Prize is AI for Science, and they are leading the frontiers of science.
Next, we delve into the secrets behind this year's Nobel Prize in Chemistry:
Proteins can be composed of anywhere from dozens to thousands of amino acids
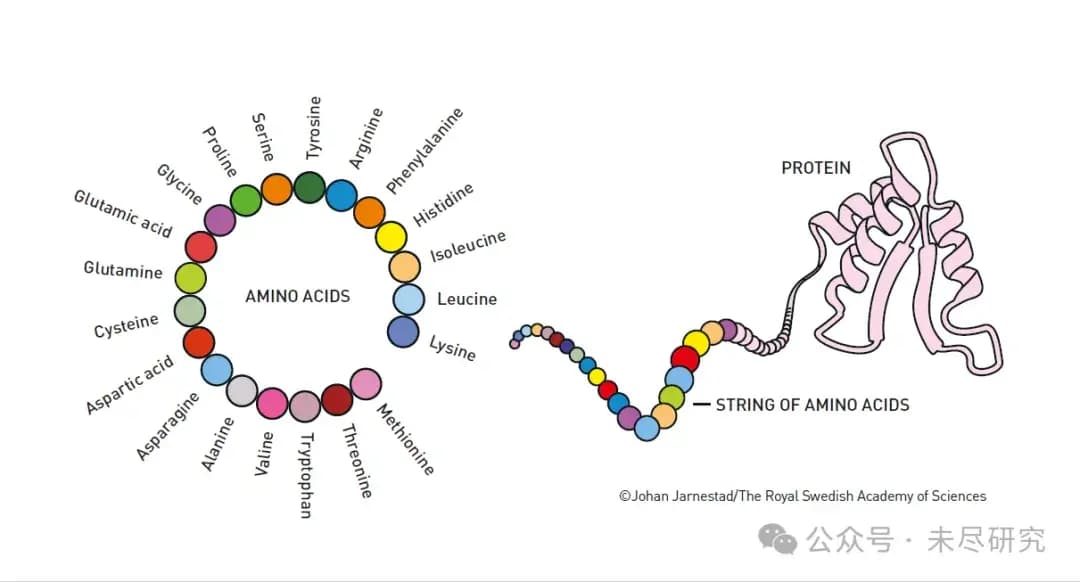
This is an educational diagram that clearly illustrates the concept of building from basic units (amino acids) to the final product (proteins).
1. On the left is a circular arrangement showing the names of the 20 basic amino acids:
a. Including Glycine
b. Glutamic acid
c. Glutamine
d. Cysteine
e. Aspartic acid
and so on...
2. On the right side, the process of forming proteins from amino acids is shown:
a. The amino acids first link together in a "string of beads" (STRING OF AMINO ACIDS)
b. This chain of amino acids eventually folds into a specific three-dimensional structure, forming functional proteins (PROTEIN)
This diagram nicely explains the two core discoveries of the 2024 Nobel Prize in Chemistry:
1. How to design new proteins using these 20 amino acids (David Baker's work)
2. How to predict what three-dimensional structure the amino acid chain will fold into (Hassabis and Jumper's AlphaFold2 work)
How does AlphaFold2 work?
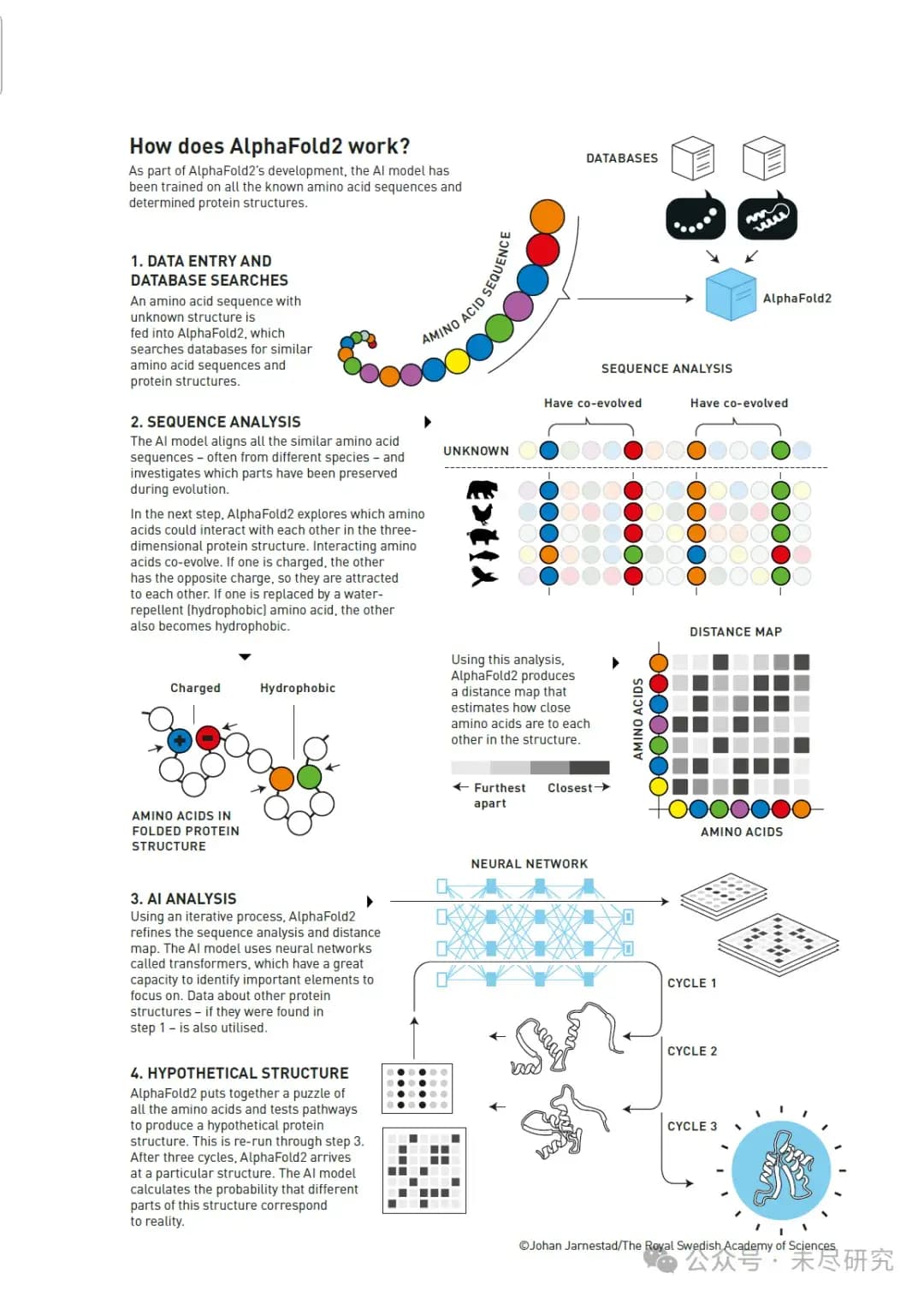
This diagram shows how AlphaFold2 combines biological knowledge, evolutionary information, and deep learning techniques to solve the 50-year-old problem of protein structure prediction.
First, as part of AlphaFold2's development, the AI model was trained on all known amino acid sequences and determined protein structures.
The workflow is divided into four main steps:
1. Data input and database search
A sequence of amino acids with unknown structure is input into AlphaFold2
The system searches for similar amino acid sequences and protein structures in the database
2. Sequence analysis
The AI model compares all similar amino acid sequences (usually from different species)
It studies which parts have been conserved through evolution
AlphaFold2 explores how amino acids interact with each other in the three-dimensional protein structure:
Charged amino acids attract each other
Hydrophobic amino acids cluster together
It generates a distance map estimating the distances between amino acids in the structure
3. AI analysis
Using an iterative process, AlphaFold2 continuously refines the sequence analysis and distance map.
The AI model uses neural networks known as transformers.
These networks identify important elements and use additional protein data from the first step.
4. Hypothesis structure generation
AlphaFold2 stitches together all the amino acids into a complete structure.
It tests different pathways over three cycles.
Finally, it arrives at a specific structure.
The AI model calculates the likelihood that different parts of the structure match the actual protein structure.
The diagram also illustrates some important visualizations:
Co-evolutionary patterns in sequence analysis
Distance maps showing the spatial relationships between amino acids
A diagram of the neural network
How multiple iterative cycles gradually refine the final structure
Top7: The First Human-Designed Protein Structure
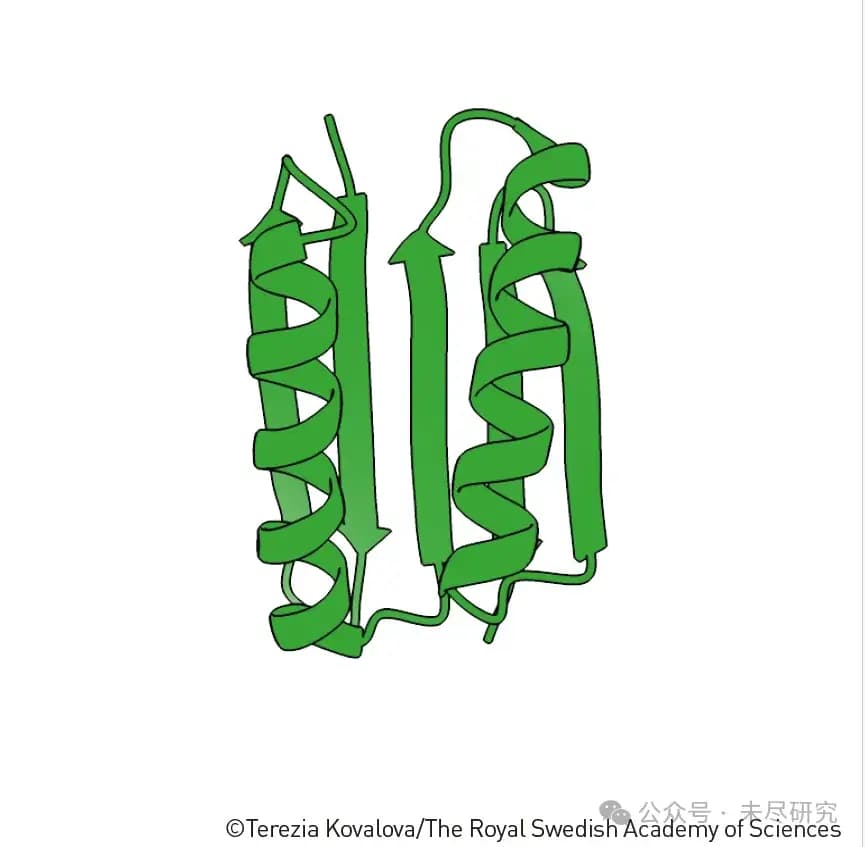
The uniqueness and historical significance of this structure are as follows:
1. Entirely New Design
This is the first de novo designed protein structure.
It was not based on any existing natural protein modification or imitation.
David Baker's team designed this structure in 2003, pioneering the era of artificial protein design.
2. Structural Features
It incorporates a novel combination of alpha helices and beta sheets.
This arrangement had never been observed in nature before.
Though it uses common secondary structure elements (alpha helices and beta sheets), their spatial arrangement is entirely new.
3. Breakthrough Significance
It demonstrated that we can design protein structures that do not exist in nature.
It showed that protein design doesn't have to be limited to mimicking natural proteins.
This opened the door to limitless possibilities in artificial protein design.
4. Methodological Significance
It validated the feasibility of computer-assisted protein design.
It laid the foundation for more complex protein designs to follow.
It established a complete workflow from theoretical design to actual synthesis.
This achievement is akin to creating the first "synthetic element" in the periodic table of protein design, much like the synthetic elements in Mendeleev's periodic table, demonstrating that humans can not only understand nature but also create entirely new things that do not exist in nature.
Proteins Developed Using Baker's Rosetta Program
This timeline showcases some of David Baker's team's key achievements in designing artificial proteins:
2016: Novel Nanomaterials
This involved designing a complex structure capable of spontaneously assembling up to 120 proteins.
The diagram shows a large spherical structure made up of purple and light green protein units.
2017: Fentanyl Detection Proteins
Designed to bind fentanyl, an opioid drug.
The diagram shows a green protein structure with a purple area that binds to fentanyl.
This protein could be used to detect fentanyl in the environment.
2021: Flu Vaccine Nanoparticles
The yellow core represents the nanoparticle.
The green outer layer mimics proteins on the surface of the flu virus.
It has been shown to work as a flu vaccine in animal models.
2022: Molecular Motor Proteins
Designed proteins that act as molecular motors.
The diagram shows a green complex structure.
2024: Geometrically Shaped Proteins
Designed proteins with specific geometric shapes.
These proteins can change shape in response to external stimuli.
They can be used to create microsensors.
This diagram illustrates the rapid development of artificial protein design, from relatively simple structure designs to proteins with specific functions (such as vaccines, sensors), and even to intelligent proteins that dynamically respond to external stimuli. These advances highlight David Baker's outstanding contributions to the field of computational protein design.
Protein Structures Predicted Using AlphaFold2
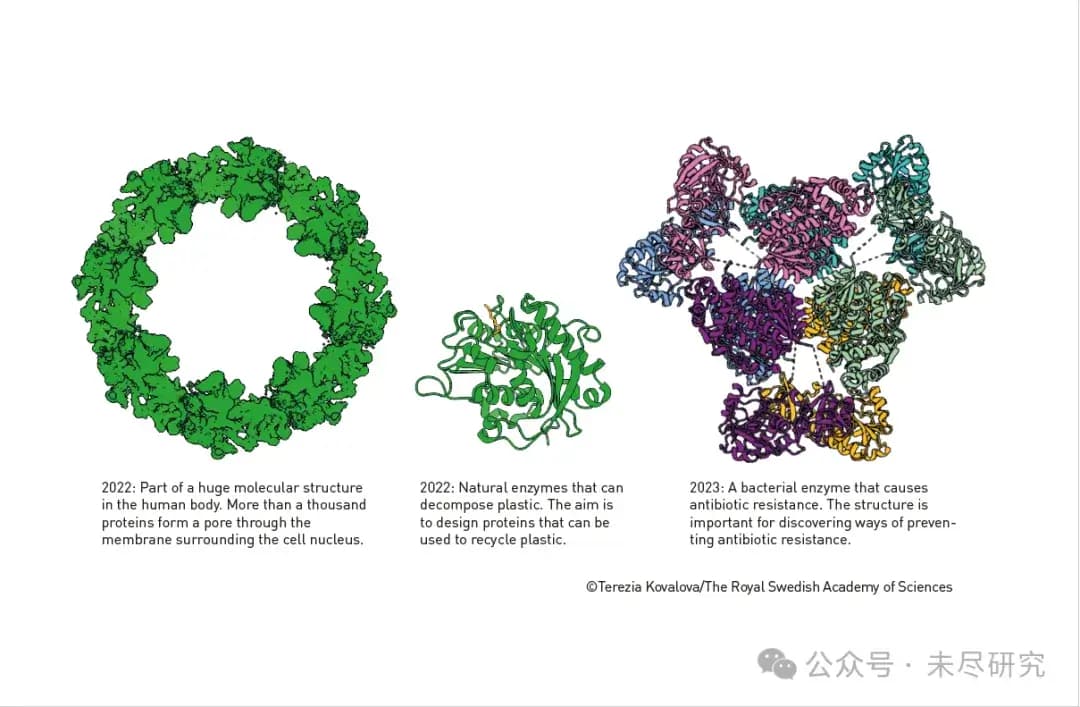
This diagram shows three important protein structures predicted using AlphaFold2:
2022: Part of the Nuclear Pore Complex Structure
Shown as a green ring-shaped structure.
This is part of a large molecular structure in human cells.
Over a thousand proteins make up a channel that crosses the nuclear membrane.
This pore is critical for material exchange between the nucleus and the cytoplasm.
2022: Natural Enzyme for Breaking Down Plastics
The diagram shows a green single protein structure.
This natural enzyme can break down plastics.
The goal of studying this enzyme's structure is to design artificial proteins for plastic recycling.
This is significant for addressing the global plastic pollution problem.
2023: Bacterial Enzyme that Causes Antibiotic Resistance
The diagram shows a complex multi-colored structure, with multiple different protein subunits.
The diagram shows a complex multi-colored structure, with multiple different protein subunits.
This is an enzyme responsible for causing bacteria to develop antibiotic resistance.
Understanding this enzyme's structure is crucial for finding ways to prevent antibiotic resistance.
This is vital for addressing the global public health threat posed by antibiotic resistance.
This diagram illustrates AlphaFold2's ability to predict complex protein structures and how these predictions can address important scientific and societal problems. From basic biological processes like nuclear pore complexes, to environmental issues like plastic degradation, to medical challenges like antibiotic resistance, protein structure prediction offers wide-ranging applications.
Following yesterday's Nobel Prize in Physics awarded to Hopfield and Hinton for their contributions to AI's foundational theories, the Chemistry Prize was awarded to Baker, Hassabis, and Jumper for using AI to design and predict protein structures. Some say physics no longer exists, while others claim chemistry is riding on the AI trend. Both are wrong. What this signifies is the profound transformation AI is bringing to scientific discovery: the Physics Prize represents "Science for AI," while the Chemistry Prize represents "AI for Science." Together, they are leading the frontier of science.